Table Of Contents
B.Tech AKTU Quantum Book will take you deep into the field of Data Analytics. To flourish in this dynamic sector, access important notes, repeated questions, and helpful insights. Unit-2 Data Analysis
Dudes 🤔.. You want more useful details regarding this subject. Please keep in mind this as well. Important Questions For Data Analytics: *Quantum *B.tech-Syllabus *Circulars *B.tech AKTU RESULT * Btech 3rd Year * Aktu Solved Question Paper
Q1. What are the various types of regression analysis techniques ?
Ans. Various types of regression analysis techniques:
- 1. Linear regression: Linear regressions are based on the assumption that the predictors (or factors) and the target variable have a linear relationship.
- 2. Non-linear regression: Non-linear regression allows modeling of non-linear relationships.
- 3. Logistic regression: When our target variable is binomial, logistic regression comes in handy (accept or reject).
- 4. Time series regression: Based on previous time ordered data, time series regressions are used to forecast future behaviour of variables.
Q2. Write short notes on Bayesian network.
Ans.
- 1. Bayesian networks are a sort of probabilistic graphical model that employs Bayesian inference to compute probability.
- 2. A Bayesian network is a directed acyclic graph with each edge representing a conditional dependency and each node representing a distinct random variable.
- 3. Bayesian networks use edges in a directed graph to model conditional dependency.
- 3. By utilizing these linkages, one may rapidly execute inference on the random variables in the graph.
- 4. By taking advantage of conditional independence, we may generate a compact, factorised representation of the joint probability distribution using the relationships provided by our Bayesian network.
- 5. Technically, if an edge (A, B) connects random variables A and B in the graph, it signifies that P(B|A) is a factor in the joint probability distribution, and we must know P(B|A) for all values of B and A in order to conduct inference.
- 6. In the Fig. since Rain has an edge going into WetGrass, it means that P(WetGrass | Rain) will be a factor, whose probability values are specified next to the WetGrass node in a conditional probability table.
- 7. Bayesian networks satisfy the Markov property, which states that a node is conditionally independent of its non-descendants given its parents. In the given example, this means that
P(Sprinkler | Cloudy, Rain) = P(Sprinkler | Cloudy)
Since Sprinkler is conditionally independent of its non-descendant, Rain, given Cloudy.
Q3. Explain the application of time series analysis.
Ans. Applications of time series analysis:
- 1. Retail sales:
- a. A clothes merchant wants to anticipate future monthly sales for several product lines.
- b. These estimates must take seasonal features of the customer’s purchase habits into account.
- c. A suitable time series model must account for varying demand over the calendar year.
- 2. Spare parts planning:
- a. To maintain an adequate supply of parts to fix consumer products, companies’ service groups must estimate future spare part demand. Thousands of separate part numbers are frequently found in parts inventories.
- b. Complex models for each part number can be created to estimate future demand utilizing input variables such as expected part failure rates, service diagnostic efficacy, and forecasted new product shipments.
- c. Time series analysis, on the other hand, can produce reliable short-term estimates based solely on historical spare part demand history.
- 3. Stock trading:
- a. Pairs trading is a practise used by some high-frequency stock traders.
- b. A strong positive correlation between the prices of two equities is utilized to spot a market opportunity in pairs trading.
- c. Assume that the stock prices of Company A and Company B move in lockstep.
- d. Time series analysis can be used to compare the stock values of various companies over time.
- e. A statistically higher than expected price difference implies that it is a good time to buy Company A stock and sell Company B shares, or vice versa.
Q4. Explain rule induction.
Ans.
- 1. Rule induction is the process of deducing if-then rules from a dataset in data mining.
- 2. These symbolic decision rules explain an intrinsic relationship between the dataset’s properties and class labels.
- 3. Intuitive rule induction underpins many real-life situations.
- 4. Rule induction provides a sophisticated classification approach that is simple to understand for end users.
- 5. It is utilized in predictive analytics to categorize unknown data.
- 6. Rule induction is often used to characterize data patterns.
- 7. The simplest technique to derive rules from a data collection is to use a decision tree built on the same data set.
Q5. Define fuzzy logic and its importance in our daily life. What is role of crisp sets in fuzzy logic ?
Ans.
- 1. Fuzzy logic is a computing approach focused on “degrees of truth” rather than “true or false” (1 or 0).
- 2. Fuzzy logic encompasses the extreme situations of truth, 0 and l, as well as the different states of truth in between.
- 3. Fuzzy logic enables the incorporation of human judgements into computing problems.
- 4. It provides an effective method for resolving numerous criterion conflicts and better assessing options.
Importance of fuzzy logic in daily life:
- 1. Fuzzy logic is required for the development of human-like AI capabilities.
- 2. It is used to create intelligent systems for decision making, identification, optimisation, and control.
- 3. Many persons interested in research and development, such as engineers, mathematicians, computer software developers, and researchers, find fuzzy logic incredibly beneficial.
- 4. Fuzzy logic has been employed in a wide range of applications, including facial recognition, air conditioners, hoover cleaners, weather forecasting systems, medical diagnostics, and stock trading.
Role of crisp sets in fuzzy logic:
- 1. It contains the precise location of the set boundaries.
- 2. It provides the membership value of the set.
Q6. Compare and contrast classical logic and fuzzy logic.
Ans.
S. No. | Crisp (classical) logic | Fuzzy logic |
1. | An element in classical logic either belongs to or does not belong to a set. | Fuzzy logic allows for a more flexible understanding of set membership. |
2. | Crisp logic is built on a 2-state truth values (True/False). | Fuzzy logic is built on a multistate truth values. |
3. | The statement which is either ‘True’ or ‘False’ but not both is called a proposition in crisp logic. | A fuzzy proposition is a statement which acquires a fuzzy truth value. |
4. | Law of excluded middle and law of non-contradiction holds good in crisp logic. | Law of excluded middle and law of contradiction are violated. |
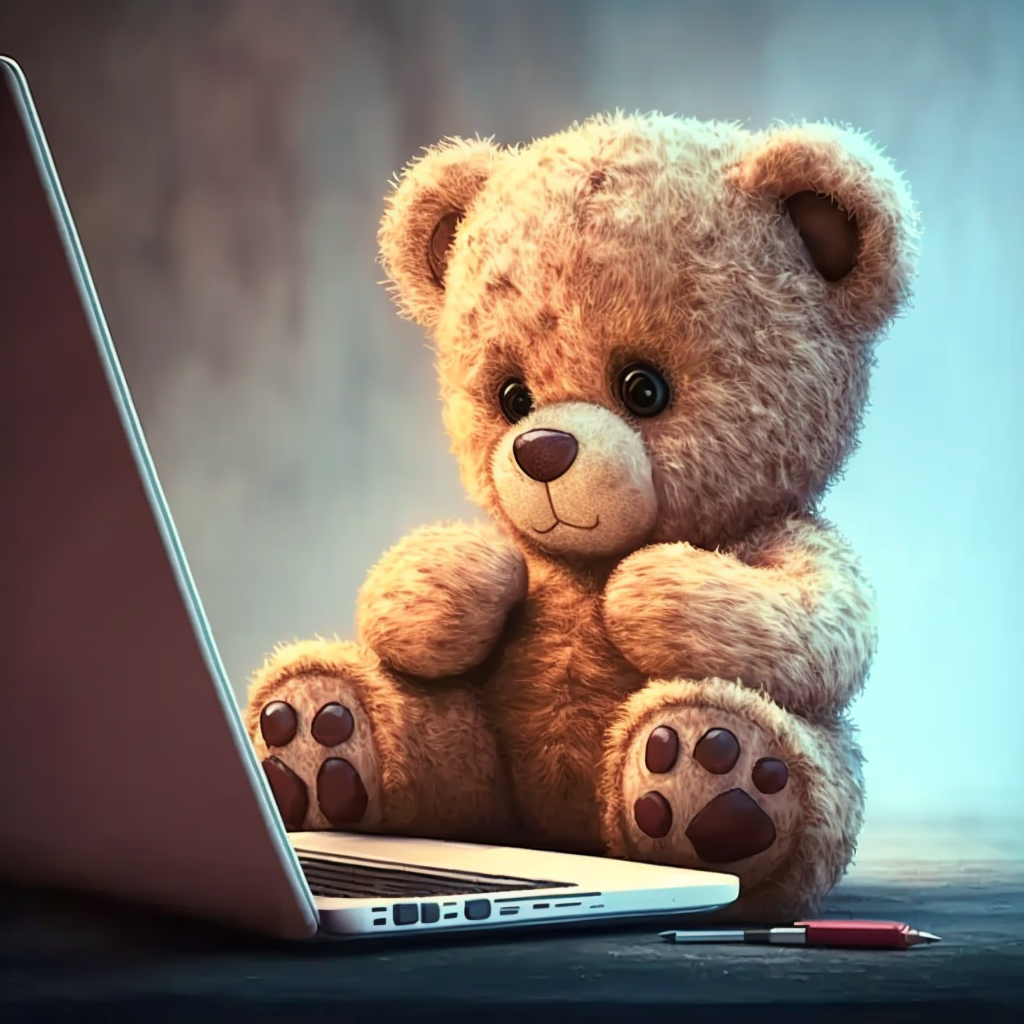
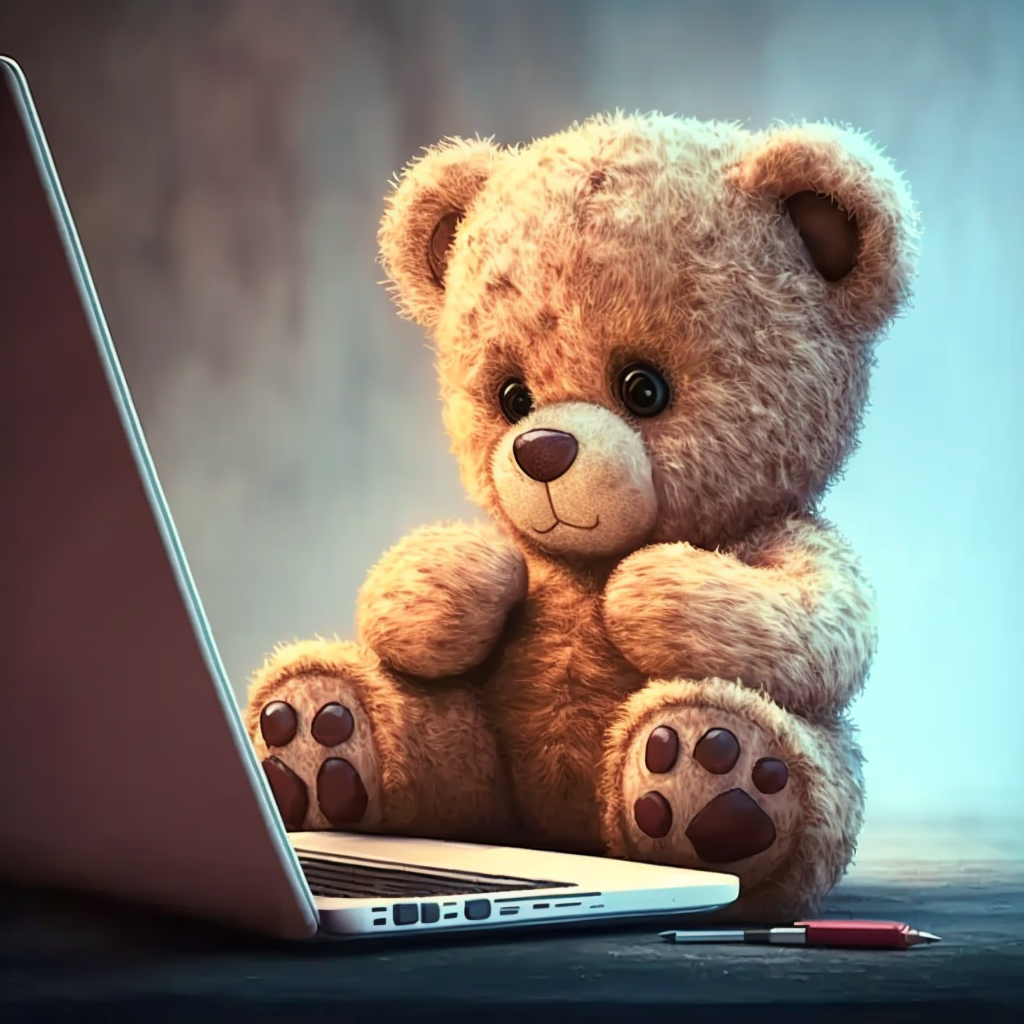
Important Question with solutions | AKTU Quantums | Syllabus | Short Questions
Data Analytics Btech Quantum PDF, Syllabus, Important Questions
Label | Link |
---|---|
Subject Syllabus | Syllabus |
Short Questions | Short-question |
Question paper – 2021-22 | 2021-22 |
Data Analytics Quantum PDF | AKTU Quantum PDF:
Quantum Series | Links |
Quantum -2022-23 | 2022-23 |
AKTU Important Links | Btech Syllabus
Link Name | Links |
---|---|
Btech AKTU Circulars | Links |
Btech AKTU Syllabus | Links |
Btech AKTU Student Dashboard | Student Dashboard |
AKTU RESULT (One VIew) | Student Result |